Having already added a 2nd P100 to the other GPU server, it was time to maximize the 2nd R720 empty PCI slot and add a 2nd P40. This GPU whilst old, still does pretty well, the main thing for me the amount of available NVRAM to work on larger models – now a combined 48G of NVRAM dedicated to the Tesla Generation GPU. I didn’t really have a script that could run a simple GPU/Memory stress test so about this so I could incremently make sure the memory and dual GPU function worked correctly. This is on Ubuntu 24.04, but should equally work on all CUDA based Python 3 platforms.
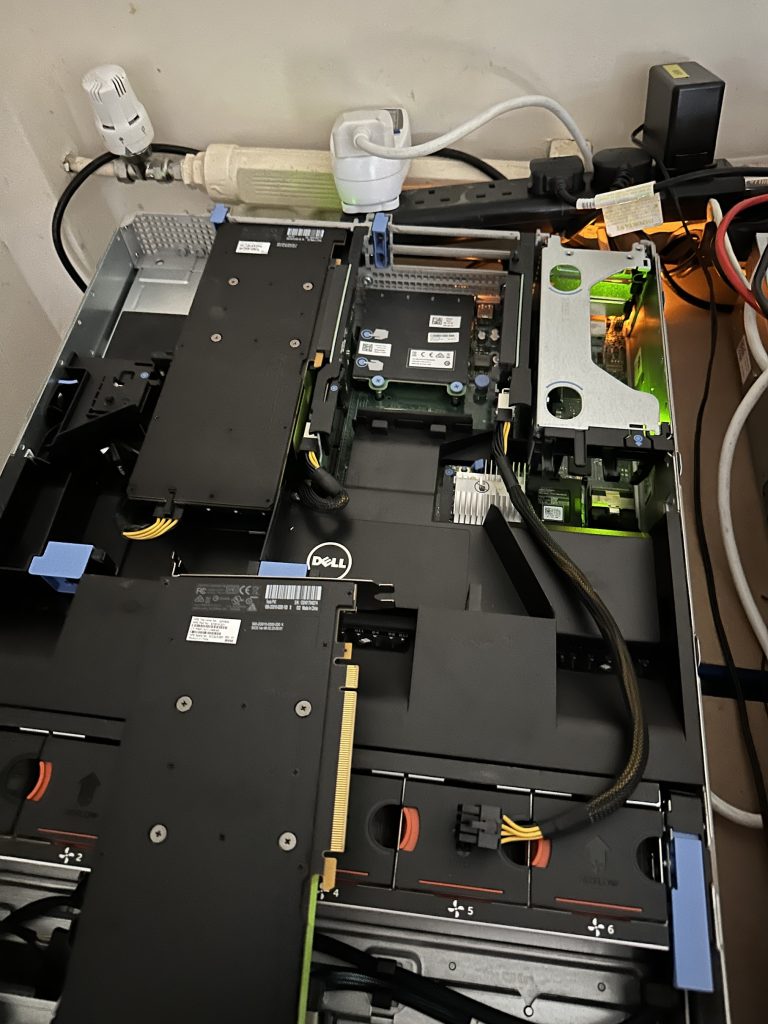
Create venv, install necessary pip packages
python3 -m venv work
source work/bin/activate
pip install torch transformers
Pytorch script to stress test available CPUs – adjust BATCH_SIZE to the amount of available memory
import torch
from torch import nn
from torch.utils.data import DataLoader, Dataset
from transformers import AutoModelForCausalLM, AutoTokenizer
# Parameters for stress test
MODEL_NAME = "gpt2" # You can use "bert-base-uncased" or another model
NUM_GPUS = torch.cuda.device_count()
BATCH_SIZE = 16 # Adjust to push GPU memory limits
SEQ_LEN = 128 # Sequence length for dummy data
STEPS = 200 # Number of steps for the stress test
# Dummy dataset for training
class DummyDataset(Dataset):
def __init__(self, size, seq_len, tokenizer):
self.size = size
self.seq_len = seq_len
self.tokenizer = tokenizer
def __len__(self):
return self.size
def __getitem__(self, idx):
text = "This is a dummy sentence for stress testing GPUs."
tokens = self.tokenizer(text, max_length=self.seq_len, padding="max_length", truncation=True, return_tensors="pt")
return tokens["input_ids"].squeeze(0), tokens["attention_mask"].squeeze(0)
# Create the model and move it to GPUs
def create_model():
model = AutoModelForCausalLM.from_pretrained(MODEL_NAME)
if NUM_GPUS > 1:
model = nn.DataParallel(model) # For multi-GPU usage
model = model.to("cuda")
return model
# Training loop
def train(model, dataloader, optimizer, steps):
model.train()
criterion = nn.CrossEntropyLoss()
for step, (input_ids, attention_mask) in enumerate(dataloader):
if step >= steps:
break
input_ids, attention_mask = input_ids.to("cuda"), attention_mask.to("cuda")
# Shift inputs for causal LM
labels = input_ids.clone()
outputs = model(input_ids, attention_mask=attention_mask, labels=labels)
loss = outputs.loss
optimizer.zero_grad()
loss = loss.mean()
loss.backward()
optimizer.step()
if step % 10 == 0:
print(f"Step {step}/{steps}, Loss: {loss.item()}")
# Main
def main():
model = create_model()
print(f"Using {NUM_GPUS} GPUs for stress test.")
tokenizer = AutoTokenizer.from_pretrained(MODEL_NAME)
if tokenizer.pad_token is None:
tokenizer.add_special_tokens({'pad_token': '[PAD]'})
model.module.resize_token_embeddings(len(tokenizer)) # Resize model embeddings to account for new token
else:
model.resize_token_embeddings(len(tokenizer))
dataset = DummyDataset(size=1000, seq_len=SEQ_LEN, tokenizer=tokenizer)
dataloader = DataLoader(dataset, batch_size=BATCH_SIZE, shuffle=True)
optimizer = torch.optim.AdamW(model.parameters(), lr=5e-5)
train(model, dataloader, optimizer, steps=STEPS)
if __name__ == "__main__":
main()
I then logged the results, adjusting the batch size until the GPUs ran out of memory, using nvtop to capture stats in real time.
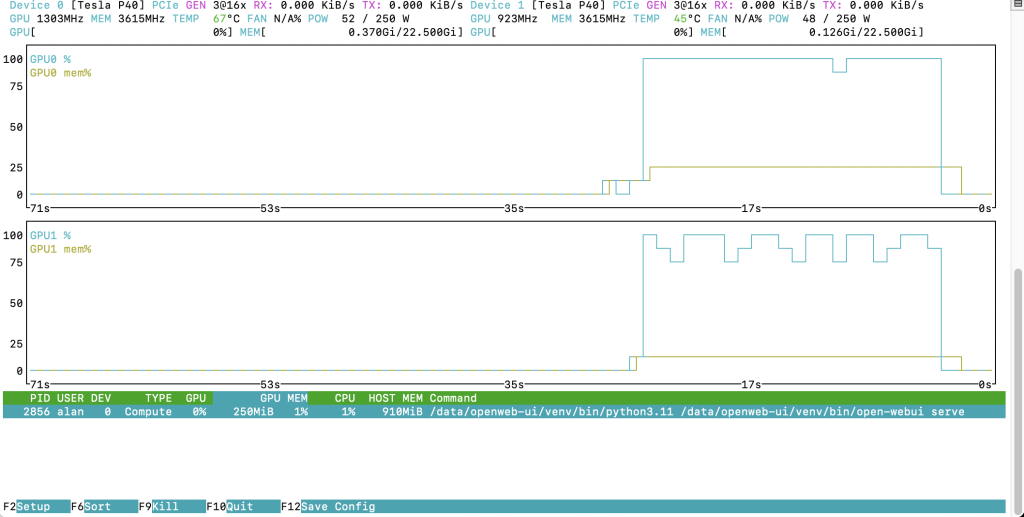
BATCH_SIZE | Memory Use max | Step Output |
16 | 8G | Step 0/200, Loss: 13.902944564819336 |
32 | 19G | Step 0/200, Loss: 14.08126449584961 |
64 | 21G | Step 0/200, Loss: 14.005016326904297 |
128 | OOM | torch.OutOfMemoryError: CUDA out of memory. Tried to allocate 3.07 GiB. GPU 0 has a total capacity of 22.38 GiB of which 1.89 GiB is free. Process 2856 has 250.00 MiB memory in use. Including non-PyTorch memory, this process has 20.24 GiB memory in use. |
110 | 32G | Step 0/200, Loss: 13.981659889221191 |
115 | 34G | Step 0/200, Loss: 13.970483779907227 |
120 | OOM | torch.OutOfMemoryError: CUDA out of memory. Tried to allocate 2.88 GiB. GPU 0 has a total capacity of 22.38 GiB of which 2.16 GiB is free. |
119 | OOM | |
118 | OOM | |
116 | OOM |
Following the stress testing, I’m confident that these are both genuine NVIDIA GPU’s and will be able to handle the workloads I use them for ! I’m doing some more ‘lab tidying’ and will take thru the lab in upcoming post.